Data is an extraordinary tool that can help marketers better understand their customers and predict their behavior. Armed with all this data, marketers can specifically tailor their direct marketing efforts to the right kinds of people, significantly increasing their chances of a sale.
Direct marketing refers to how we directly communicate with target audiences, delivering messages via direct channels like social media, phone calls, and email campaigns. So much data can be accrued from this kind of marketing, and machine learning is a crucial tool for managing this substantial data.
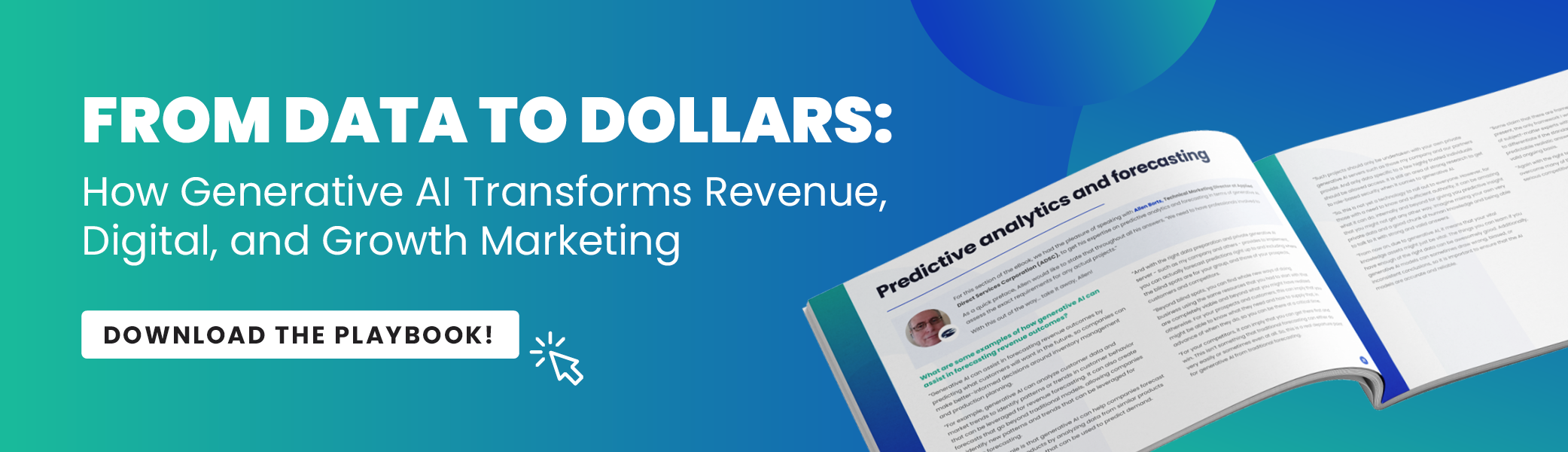
Machine learning is a subset of AI that uses data to answer questions. One of the key benefits of machine learning is its ability to “learn” so it can make predictions or decisions without being specifically programmed to do so. 56.5% of marketers are using machine learning for content personalization. It can be used to learn the patterns of website users and predict their future behavior.
Using these behavioral and demographic clues, it can devise individual predictions for how likely a consumer is to buy at that moment.
In this article, we’re going to take a closer look at:
- Why you should be using machine learning in your marketing
- Rule-based segmentation vs machine learning
- Main applications for machine learning in marketing
- Real-life examples of machine learning in marketing
- How to improve your marketing analytics through machine learning
Why should you be using machine learning?
In the past decade, the marketing industry has been dominated by machine learning. Machine learning has proven highly beneficial to big companies such as Amazon, Google, and Facebook. 92% of companies are now using AI-driven personalization to drive growth.
Basically, ML provides you with an accurate and precise analysis of your data within minutes. No marketer wants to spend endless hours analyzing data trying to identify key patterns and what campaigns are working best for their brand. ML will do all the messy data analysis for you, so you can focus your time and energy on the tasks that you actually want to do!
Rule-based segmentation vs machine learning
Marketers have been using personalization in their direct marketing strategies for a long time and in many forms. From a “welcome back” message for returning users to more individualized product recommendations in email newsletters, it is a known fact that customers who experience a more personalized shopping experience are more likely to spend and keep returning, whether it’s B2B or B2C.
Rule-based segmentation is a more simplistic way of personalizing marketing efforts than using machine learning. It uses rules determined by “if-then” scenarios, such as if it detects a website user from a specific location, it shows nearby events to that person. Or it might divide users by demographics such as age and gender and push marketing campaigns geared towards those groups.
But using rule-based systems takes a lot of work to add in all those variables manually, and many incorrect assumptions are made about customers. Marketers may pick a variable they believe to have the most significant impact on decision-making when it might actually be far from reality. With all the data marketers have access to, more advanced techniques are needed to use it to its full potential.
Personalized marketing is now considered a standard service, with 72% of consumers claiming they only engage with personalized messaging, so it’s only a matter of time until it becomes the norm for B2B too.
Enter machine learning. You feed the algorithm your data which is then used to determine patterns. This type of marketing goes far beyond rule-based personalization, as the algorithm can define segments in a way that humans simply can’t or wouldn’t have time to.
Machine learning can analyze each traffic segment and provide the most relevant content to different audience groups. As a result, personalization can exist for every single visitor, and it can be done automatically.
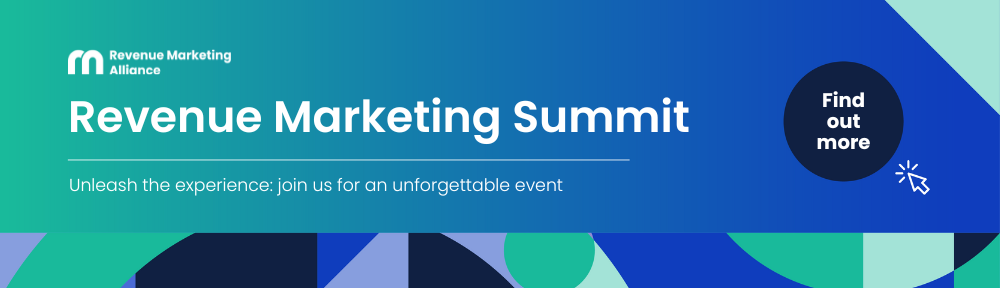
Machine learning applications in direct marketing
So we’ve seen in a nutshell what machine learning can do for marketing personalization, so here’s a closer look at the specifics of how marketers can use machine learning in their campaigns.
Predicting customer lifetime value
Customer lifetime value (LTV) is essentially looking at the worth of your customer base and identifying which customers are going to stay with you the longest.
Usually, LTV is calculated after the customer has left by looking at the total profit you have gained from the customer and the amount of time they have been with you.
If you use ML you can predict LTV before the customer leaves you. The right type of algorithm can analyze the behavior of each one of your customers to give an accurate prediction of their lifetime value. This information allows you to direct your marketing and advertising campaigns to the customers who are likely to stay with you for longer.
Improving customer service
ML is a highly intelligent application that can greatly enhance the service provided to your customers. 75% of businesses that use artificial intelligence and machine learning have seen an increase in customer satisfaction by more than 10%, which is pretty great!
Chatbots are a key application of ML for improving customer service. They provide instant answers 24/7 and have the ability to pass the customer over to a human operator if needed. You can also use chatbots for outbound marketing by sending automated messages to customers once they’ve purchased recommending products that they might be interested in.
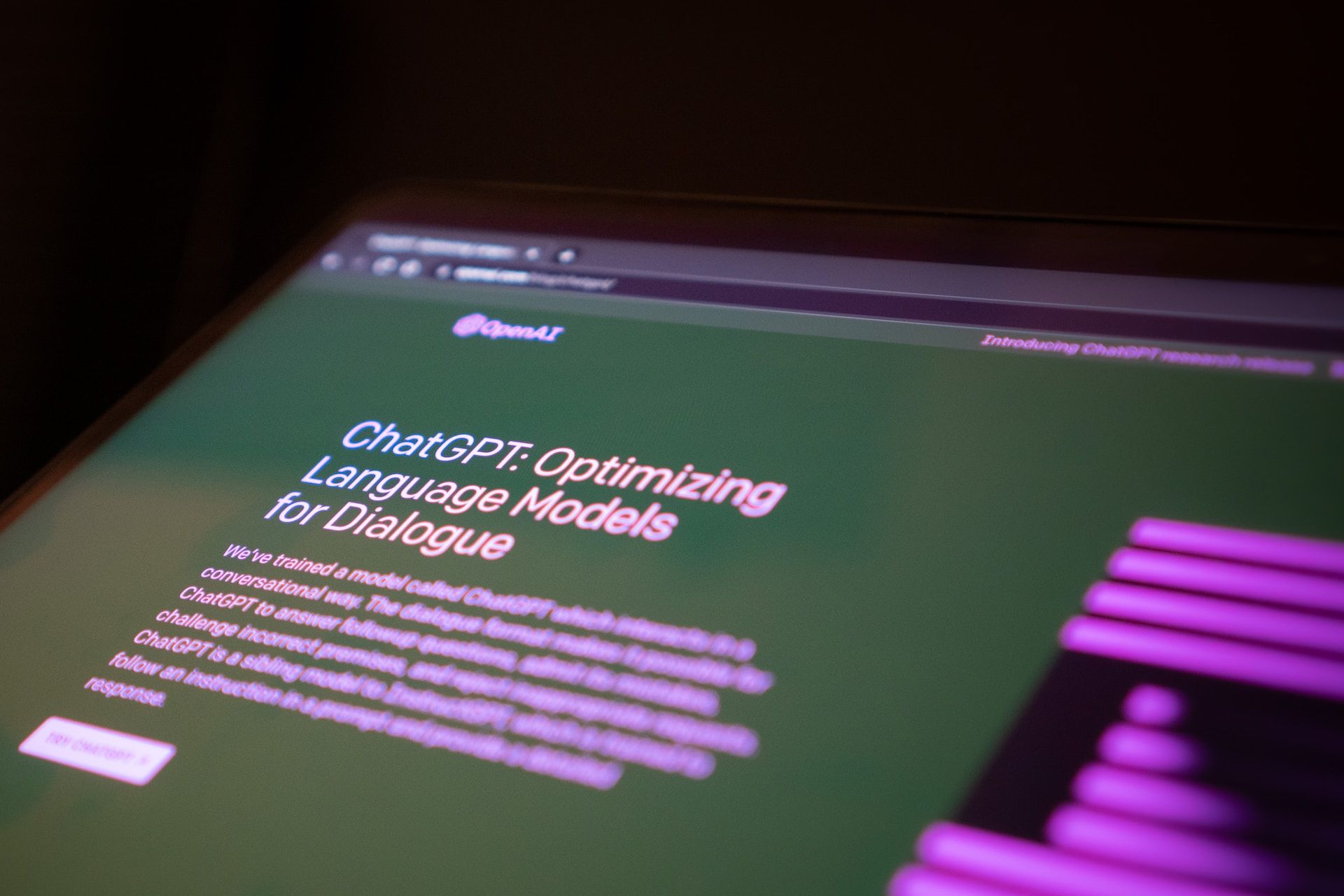
Predictive targeting
ML can be used to ensure your budget is being spent on the right people: the people who are highly likely to purchase your product/service.
ML uses an algorithm to create an ideal customer profile, based on the behaviors of your current customers such as their interactions with your website and the type of content they have engaged with the most, as well as the demographics of their business such as company size and industry.
This allows you to identify a target audience that has a high probability of buying your product/service. You can then target your ads to this specific audience increasing both your ROI and revenue.
Lead scoring
Wasting time and energy on leads that are never going to be converted into a sale is something that we all hate doing. Luckily by using ML, you can reduce the chances of this happening.
Propensity models are great for scoring leads based on the probability of them making a purchase. Without ML, these models are often developed by importing data into spreadsheets and then analyzing it, which is time-consuming and often very ineffective.
ML can generate these propensity models for you within minutes and give you accurate results about the probability of each lead buying a product/service you offer. This means your sales team can focus their time on the leads with the highest probability of being converted into an actual sale!
It’s important to note that the more data you have, the more effective and accurate the algorithm becomes. It’s recommended to have at least 300 customers that have already brought from you to have enough data to generate the most accurate probabilities of leads.
Predicting churn
Predicting when and why customers are going to leave your brand will allow you to reach out and offer a solution to their problem or an incentive to stay, like discounts, reducing the chance of churn.
However, an inaccurate prediction can send you on the dangerous path of giving the wrong customers discounts, meaning you will not only fail to retain the correct customers but also lose revenue.
ML can reduce the chance of this by providing you with an accurate and well-analyzed model that looks at customer behavior to work out which customers are most likely to churn. You can also identify what factors are causing the customers to leave allowing you to adjust your product/service accordingly to keep your churn rate low.
Personalization
Personalizing your content to meet the individual needs of each customer is a vital part of your marketing strategy. It can give you the upper hand when a customer is deciding to buy from your brand or not. Research shows that more than half of customers will decide to go with a competitor if personalization isn’t part of their journey with a brand!
Machine learning can easily personalize your content for each of your customers. It can send personalized emails, create a personalized homepage, and generate offers that are relevant to your customers’ needs based on their behaviors and past purchases.
Product recommendations
Recommending products/services through ads is more effective than just waiting for customers to find you organically. In fact, 91% of smartphone users who bought or who are planning to purchase a product have said that it was after seeing an ad that was relevant to them.
Tools such as TensorFlow and Google Cloud use ML to create recommendation algorithms based on customer behaviors. They can also recommend to you what type of products the market is currently demanding so you can tailor your current and future products/services to meet that specific demand.
Marketing automation
Marketing automation should be incorporated into every brand’s strategy. There is a 451% higher rate of qualified leads in brands that use marketing automation tools to deal with customer experience than brands that don’t!
And yet again, machine learning is the gold dust that will enable you to easily and effectively automate all of your marketing campaigns from Google ads to weekly email newsletters to discount systems.
Something like SALESmanago Copernicus is a great tool to use to automate your marketing. It will analyze your customer data and automate your marketing to provide personalized content tailored to the specific needs of your customers.
Create and optimize your content
Do you struggle to come up with content for your brand? Not sure which type of content is working best for attracting new customers and retaining current ones?
ML can be used to research and identify which types of content are performing well at the moment for you and can also recommend content that is working well for your competitors. You can also use ML to carry out A/B testing, meaning you can try out various types of content and see which one is working the best by looking at the resultant data.
ML tools like Phrase and Frase.io are great for content creation and optimization.
Phrase can write engaging content for email campaigns and push notifications and Frase.io can help with your SEO strategy. It uses ML to analyze your current content and tell you exactly what you need to do in order to rank higher on search engine results pages.
You can also curate your content using ML. Curata and Vestorly are two really useful tools that will allow you to do this. They will send the right type of content to the right type of person at the right time meaning the probability of the customer purchasing is high helping you to increase your ROI.
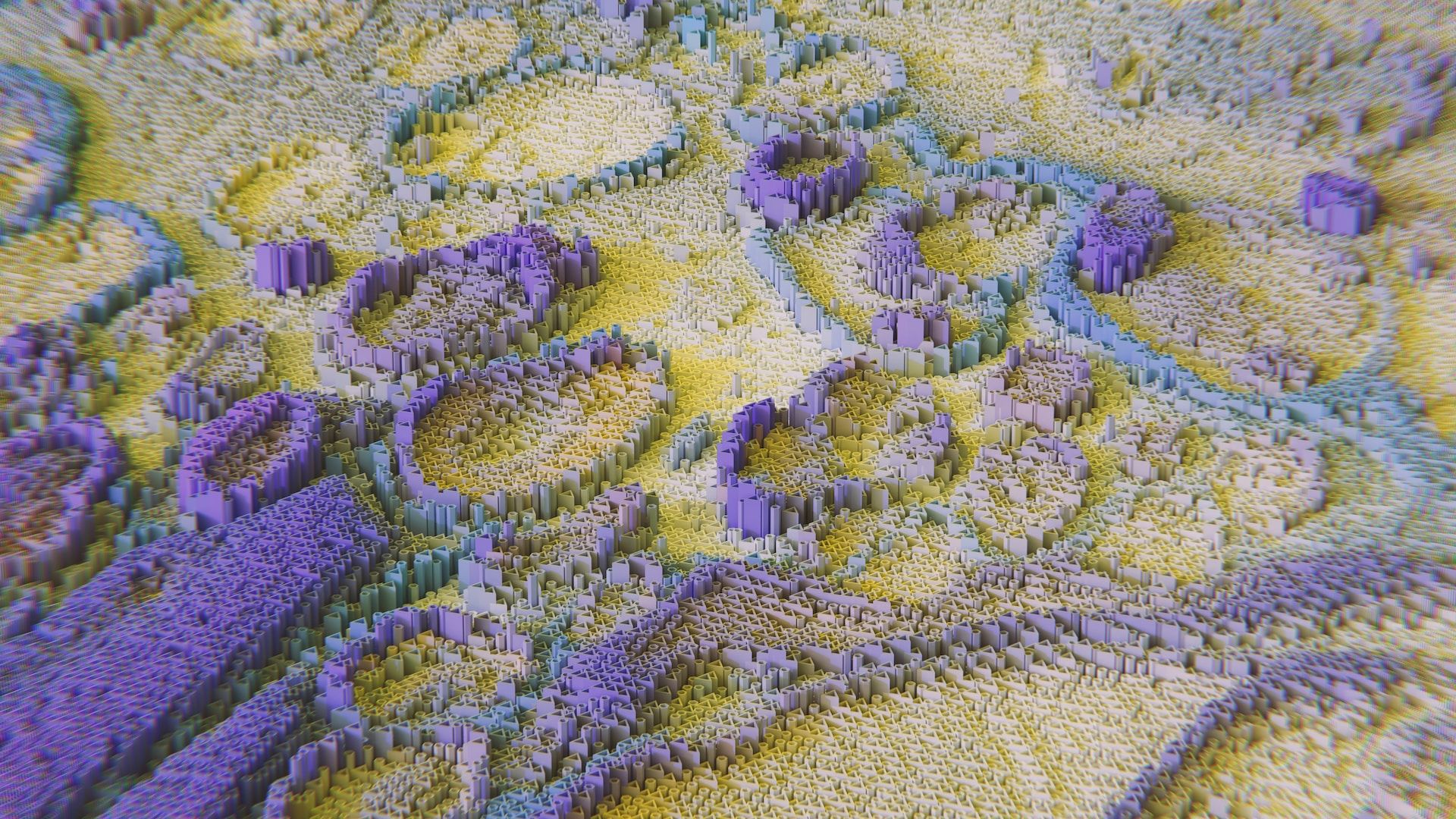
Case studies
Still interested to learn more? Here are some real-life examples of how machine learning is being used right now in the B2C world to inspire us B2B marketers:
Netflix
As the world’s biggest streaming service, Netflix has over 232.5 million subscribers in 190 countries, which is an awful lot of data to work with. Netflix invests heavily in machine learning to improve its recommendation algorithms for users. Machine learning helps shape what programs and films are shown to specific users, giving a personalized experience to every customer.
eBay
The e-commerce platform eBay utilizes machine learning to help them bypass the language barriers of their users. To connect international buyers and sellers on the platform, it brought in a machine learning system to translate product titles, descriptions, and reviews.
This kind of system increased their overall commerce, and eBay’s Senior Manager of Machine Translation Science has said that it can “connect global customers” to provide the best experience.
Amazon
Amazon has been a big proponent of machine learning for many years to better understand its customers and provide personalized shopping recommendations. But more recently, the e-commerce giant has been using algorithms to make more sustainable packaging decisions.
Using product data and customer feedback, Amazon implemented a machine-learning system to eliminate waste by identifying products that can be shipped in paper bags or folded into smaller packages.
Improving your marketing analytics through machine learning
Marketing analytics is the study of data gathered from marketing campaigns to determine if your marketing efforts are working and to optimize future campaigns. In this section, we’re going to look at how machine learning can be used to improve your marketing analytics to achieve the best results.
Customer segmentation
Customer segmentation has long been a tool in every marketer's belt. It divides customers into sub-groups typically based on some shared characteristic, such as gender or age (mainly in B2C), or by company type or industry (for us B2B cool kids), allowing marketers to serve targeted content to those groups.
It’s a basic way of personalizing your marketing efforts. But it’s often done manually, which can be a very time-consuming process, not to mention inaccurate if the marketer is unknowingly choosing the wrong metrics to focus on.
Machine learning algorithms can detect similarities within your customer base and automatically and much more accurately group them together. It measures customer activities on your website to create more personalized and specific metrics to group your prospects.
While a marketer may look at a few broad metrics, a machine can look at many more metrics in mere seconds and apply them to your marketing efforts. It can look at all website users across certain time periods and predict, based on previous behaviors, which users are likely to make a purchase and when.
With all this data, marketers can craft the perfect campaign that reaches their customers at exactly the right time. The algorithm will cluster audiences into segments based on real-world data rather than general assumptions for more effective targeting and messaging, providing an overall improved ROI.
Improved depth of data
Data is a crucial tool for marketers, but they can often be limited by their ability to access and analyze it. Gathering and analyzing data manually is a time-consuming and labor-intensive process, which can often be inaccurate because data changes and updates so quickly.
Machine learning can provide marketers with large, up-to-date data sets that they can use to obtain deeper insights, such as predicted next steps, helping you to understand your consumer behavior and your campaign performance.
By diving into deep data, you can better develop personalized marketing strategies for the highest possible ROI. This kind of data not only reveals your customers’ preferences but also helps you to establish which channels are the best for engaging them.
With machine learning, marketers can respond more quickly to changes in the quality and quantity of customers brought in by marketing campaigns. All of this can be done in real-time, and you can adapt to any changes or new data, improving the overall quality of your data analysis in less time.
Predictive analytics
Predictive analytics uses data and machine learning to predict future events. For marketers, this can be used to better understand which campaigns are working and which will lead to an increase in sales in the future. Almost half of B2B marketers are currently using predictive analytics for customer insights.
It links to customer segmentation, providing insights to consumers based on their past behaviors so they can be sent targeted messaging at the right time. With predictive analysis, marketers can determine where to focus their marketing efforts based on the predicted value of a customer.
Predictive data identifies the right kind of advertising channels and the right times to increase marketing spending or reduce investments.
It can help you qualify and prioritize leads, allowing you to devote more attention and spend on the right prospects rather than wasting it on those that won’t respond to marketing.
Predictive analytics can also help retain customers, which is essential for marketers as it’s five times more expensive to gain a new customer than it is to retain one. It helps you better understand your customers’ needs, providing you with upselling opportunities to keep them spending in the future.
Chatbot analysis
Chatbots are a great way to engage with customers and improve customer support, as they have the benefit of being available 24 hours a day to offer help and assistance.
In fact, chatbots have seen a 92% increase in usage since 2019, and 87.2% of consumers rate their chatbot experiences within the range of neutral to positive. As we well know, once consumers have given the thumbs up to something, it’s only a matter of time before it becomes prevalent in B2B. Machine learning significantly improves the performance of chatbots while also gathering vital data.
Machine learning uses the data of previous chatbot interactions to help it improve its responses to future customers. It can be “taught” to “talk” with human customers and respond effectively to their questions and problems. By using algorithms and conversational inferences, the chatbot can become smarter and more human-like with its responses.
Aside from improving your customer service, you can utilize chatbots as a tool to learn about your customers and adjust your service strategies accordingly. Your chatbot can record, store, and retrieve customer data better than a human can manually, providing you with a detailed analysis of trends and behaviors. With all this data, you can gain a deeper understanding of your customer base and any recurring pain points to address.
Conversion rate
A conversion rate looks at the likelihood that a customer will make a purchase. When you harness this data, you can focus your marketing efforts on those more likely to make purchases and avoid wasting time and effort on those much less likely to do so.
Increasing conversion rate with machine learning can be done in a few ways, such as predicting which version of a landing page will perform best for each visitor; using dynamic pricing to predict the best price for each user, and predicting which free users will convert into paying customers.
The algorithms use extensive data points for each user to work out the likely conversion rate of each one, classifying converters and non-converters within chosen variables. Marketers can then adjust their strategies to focus mainly on the users determined to become converters, increasing the likelihood of purchase.
Final thoughts
Machine learning has the potential to transform how we market to customers, and it will only keep getting more advanced as time goes on. You can utilize the power of machine learning to remove the repetitive, time-intensive, mundane tasks that many marketers find tedious and focus on those more critical tasks that require the human touch.
What applications are you interested in for machine learning in B2B marketing? Are you already utilizing it? Share your wins with the Revenue Marketing Alliance Community!
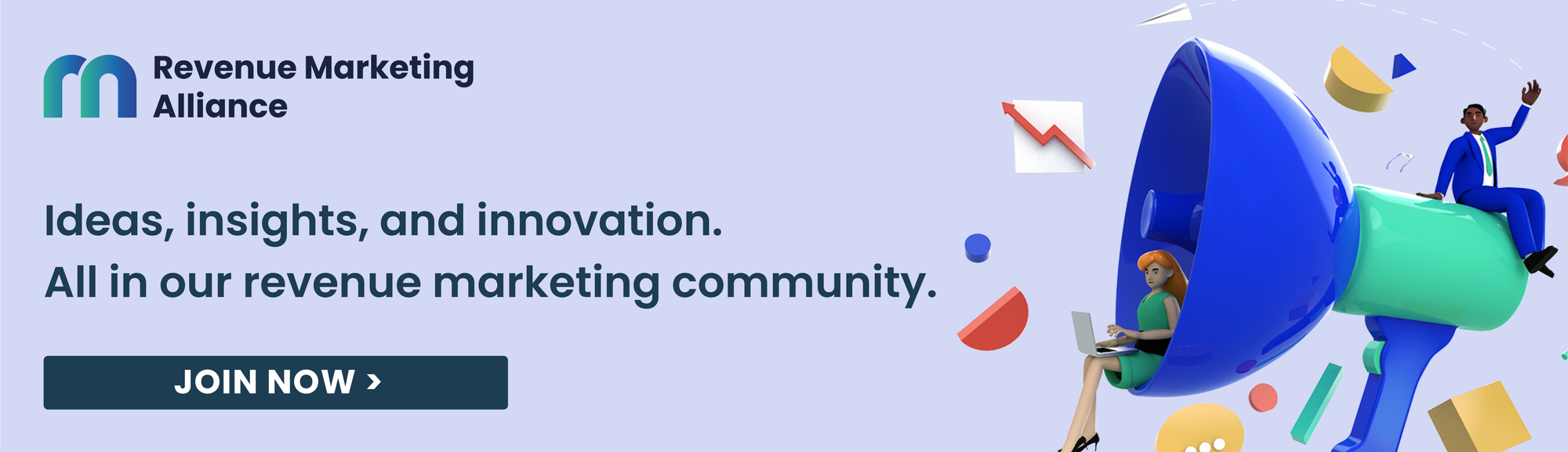