Since the dawn of advertising, marketers have been challenged to decide where to spend their ad budget to attain the best results. One of the most compelling aspects of digital advertising (beyond providing a new way to reach audiences), is the ability to directly and precisely measure the outcome of an ad. After marketers gravitated to digital, they believed they were finally poised to solve the marketer’s dilemma – and for a time, they did (sort of).
The evolution of marketing measurement
In the early days of internet advertising, you’d create an SEO-friendly website, buy some keywords, maybe set up an email campaign, and be good to go. The cost to advertise using these new channels was relatively low, and not yet saturated with competition.
Digital advertising was largely treated as a new frontier rather than a core part of most companies’ media mix. As a result, measuring the sales that came from your keyword campaigns was fairly straightforward, largely because the path to purchase was pretty simple: search, click, buy.
Fast forward to today, and the landscape has become exponentially more complex. Brands now invest significant budgets across numerous platforms, including major social networks like Facebook, Instagram, and LinkedIn, search engines such as Google and Bing, emerging channels like connected TV, and traditional media such as television, radio, and direct mail.
Modern marketing tactics have evolved to encompass sophisticated approaches including retargeting, personalized experiences, onsite chat, display advertising, and influencer marketing.
There are many numbers out there, but averages suggest that a customer makes six to eight touches before arriving at a purchase decision. The simple, linear path to conversion has become exponentially more complex. And let’s not forget that, along with these new ways to communicate with customers, traditional channels like TV, radio, and direct mail have not disappeared.
All these “touch points” now play a role in influencing a customer to convert, so which ones get the credit? The answer is all of them, to a degree.
What is RoAS (Return on Ad Spend)?
Return on ad spend (ROAS) represents the amount of revenue generated for every dollar spent on advertising. While this might seem straightforward, accurate measurement has become increasingly challenging in today's complex digital landscape. The interplay between advertising efforts and outcomes is no longer directly apparent, especially when considering awareness campaigns designed to feed other channels like display remarketing.
Traditional attribution models have significant drawbacks because they're based on heuristics or rules, rather than data-driven insights. The last-click attribution model, while most common due to its simplicity, ignores the majority of the customer's path and assigns the entire conversion value to the final touchpoint.
Similarly, first-click attribution credits only the initial marketing touchpoint, disregarding all subsequent interactions that influenced the purchase decision.
Linear attribution attempts to address these limitations by distributing conversion credit equally among all touchpoints, but this approach fails to recognize that different interactions may have varying degrees of influence on the final purchase decision.
The time decay model, which gives more credit to recent touchpoints, still relies on arbitrary rules and potentially undervalues early interactions that may have been crucial in initiating the customer journey.
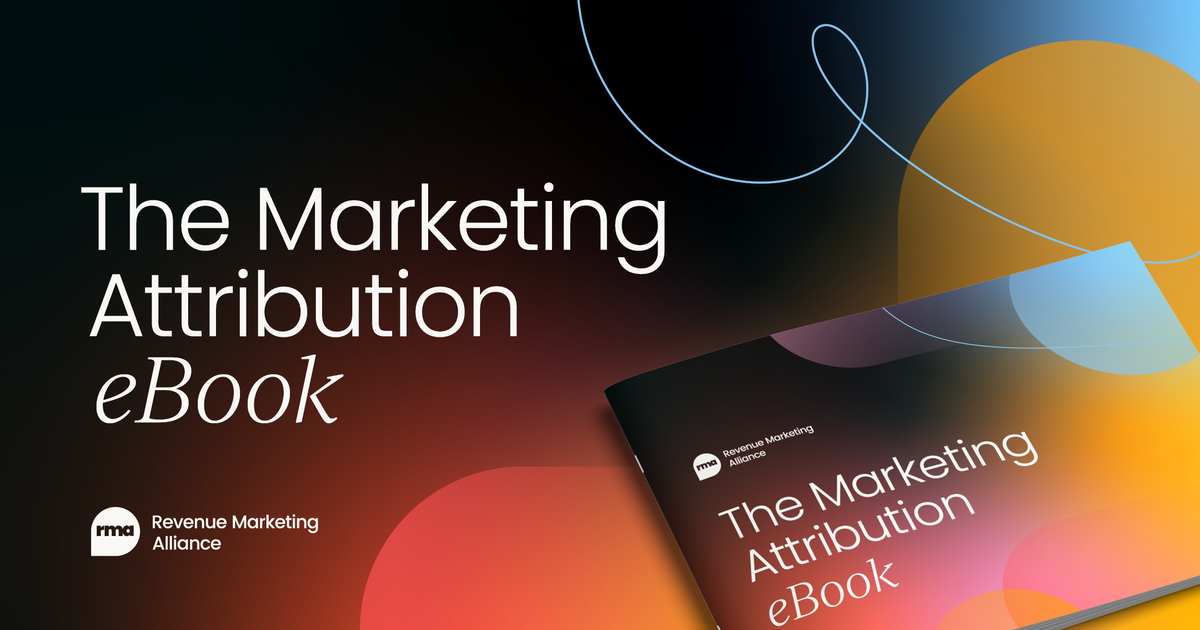
Marketing attribution models: A quick recap
Traditional attribution models all have their pros and cons; however, the biggest drawback they share is that they are all heuristic models, meaning they are based on rules or heuristics. These rules decide how to split the value of a sale among touchpoints, including…
Last click attribution
This is the most common model, probably because it’s the simplest to implement, understand, and explain to others. It ignores the majority of the customer’s path and assigns the entire conversion value to the last touch or “last click”. The shortcomings of this approach are obvious: if all the touch points before the last click are ignored, then they’re simply not being measured.
First click attribution
Like last click, this model places all the emphasis on the first piece of marketing to touch a prospective customer. It suffers from the same shortcomings, as it ignores a large portion of the customer journey.
Linear attribution
Linear attribution models distribute the conversion credit equally among all touch points along the path to purchase.
Time decay models
Time decay models distribute conversion credit based on touchpoint recency. The last click gets the most credit, followed by the second-to-last, and so on.
The above models are all poor proxies for understanding what actually drives results. They are designed around convenience and ease of use but are ineffective at determining what’s working.
The limitations of traditional marketing attribution models
The biggest problem with all these models is that heuristics are arbitrary, inflexible, and subject to bias (I’ll talk more about bias in the next installment). The models are all performance-based in that they mesh with performance advertising but fail to credit branding, awareness, and other top-of-funnel efforts.
Over the last several years, the cost per thousand ad impressions (CPM) has grown dramatically. This trend is driven by massive budget shifts to digital channels, which were in turn driven by massive shifts in how much time people spend online. As a result, brands need to be more targeted and cut the fat when it comes to unneeded ad spend.
Compounding this problem, both in-house marketing teams and external agencies often work in silos. The paid media agency, the internal social media team, our content group, and so on are often only directly aware of the channels they’re tasked with managing and measuring results in their siloed contexts.
Major challenges in measuring ROAS
The bias problem
Several types of bias can significantly impact ROAS measurement accuracy. Confirmation bias leads marketers to select data that supports their existing beliefs, potentially skewing the interpretation of KPIs and influencing which metrics receive attention. This natural human tendency can cloud marketing decisions and prevent objective analysis of campaign performance.
Correlation bias presents another significant challenge, as marketers often perceive relationships between events and outcomes even when none exist. This bias is actually built into models like last-click attribution, which assumes that the final touchpoint was the most influential simply because it occurred immediately before the purchase.
Platform bias represents a systematic challenge in the industry, as advertising platforms have an inherent tendency to claim credit for conversions due to their involvement in the customer journey.
Technical and organizational challenges
Double-counting has emerged as a significant technical challenge in ROAS measurement. When multiple platforms or agencies are involved in a customer's journey, each may claim credit for the same conversion. This results in inflated reporting that doesn't reconcile with actual sales figures, making it difficult to determine true marketing effectiveness.
The problem of siloed data compounds these challenges, as marketing data often exists in fragmented systems across different platforms and tools. Traffic analytics, sales data, and campaign information typically reside in separate systems, making it nearly impossible to get a holistic view of the customer journey without significant integration efforts.
Organizational silos present an equally challenging obstacle. Marketing teams often operate in isolation, with competing incentives that can lead to counterproductive behaviors. When teams are measured and rewarded based on their individual channel performance rather than overall marketing success, it becomes difficult to implement a truly integrated approach to ROAS measurement and optimization.
Is there a solution to the problem of ROAS measurement?
Modern attribution solutions have emerged to address the limitations of traditional approaches. Two leading methods have gained prominence in the industry: the Shapley value approach and Markov chain attribution.
Shapley value approach to attribution
The Shapley value approach, based on game theory, provides a mathematical framework for fairly distributing credit among marketing touchpoints. This method measures the marginal contribution each touchpoint makes to the conversion process, rewarding only genuine contributions while eliminating credit for passive participants. The approach is particularly valuable because it's mathematically proven to provide fair allocation and uses a transparent, well-established algorithm.
Markov chain attribution
Markov chain attribution takes a probabilistic approach, using Bayesian statistics to model customer journeys. This method excels at considering the sequential nature of touchpoints and modeling transition probabilities between different marketing channels. By simulating the removal of individual channels, Markov chains can measure true channel contribution and help optimize the overall marketing mix.
How to implement effective ROAS measurement
Successful ROAS measurement requires a comprehensive approach that addresses both technical and organizational challenges. Organizations must focus on integrating their marketing data into a centralized system that provides a single source of truth about the customer journey. This integration should encompass all marketing channels, sales data, and campaign information.
Team alignment plays a crucial role in successful implementation. Organizations need to create shared objectives that encourage collaboration rather than competition between departments. This may require restructuring incentives to reward overall marketing success rather than individual channel performance.
The technical implementation of attribution solutions requires careful consideration of the appropriate model for your organization's needs. Whether choosing Shapley value or Markov chain attribution, proper setup and ongoing maintenance are essential for accurate results.
As digital marketing continues to evolve, organizations must adapt their ROAS measurement strategies to address new challenges. Privacy regulations are becoming increasingly stringent, requiring organizations to develop robust first-party data strategies while maintaining customer trust. Technical agility is essential, as platforms and technologies continue to evolve rapidly.
Success in ROAS measurement requires a commitment to continuous learning and improvement. Organizations must stay informed about industry trends, test new approaches, and share knowledge across teams. This ongoing education ensures that marketing teams can adapt to new challenges and opportunities as they arise.
Let's recap
Accurate ROAS measurement is undoubtedly essential. By implementing advanced attribution models, addressing organizational challenges, and maintaining a data-driven approach, marketers can achieve a clear understanding of their marketing effectiveness and optimize their investments accordingly.
Success requires a combination of technical expertise, organizational alignment, and strategic thinking. By following the guidelines in this comprehensive guide, organizations can develop the capabilities needed to tackle the challenges of modern ROAS measurement and optimization, ultimately driving better results from their marketing investments.